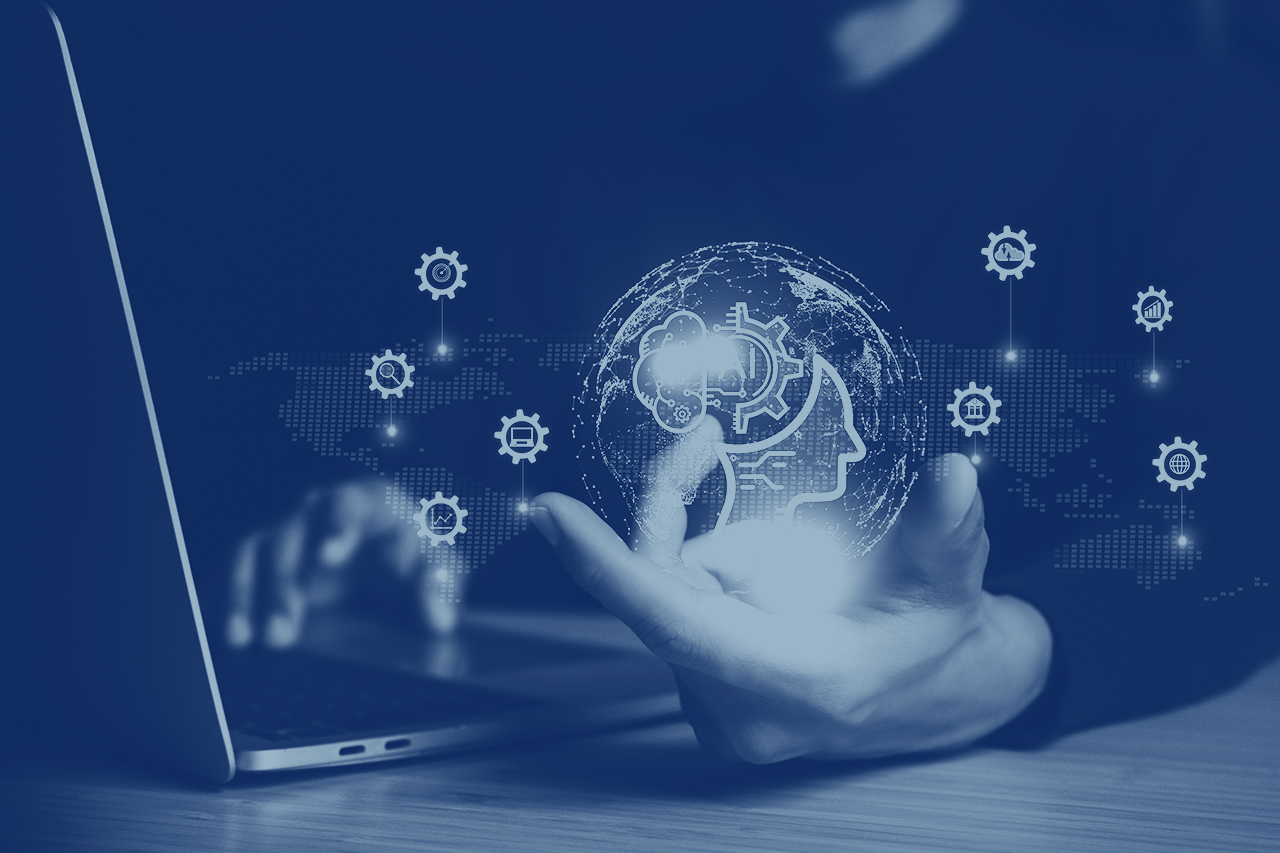
8 Ways to Mitigate ChatGPT and Generative AI Risks
ChatGPT has definitely raised the public consciousness around generative AI and large language models (LLMs). This piece explains the top risks of these tools and recommends eight methods to mitigate them prior to allowing their usage within your organization.
Business Risks of Generative AI
We see four categories of business risk inherent with using generative AI — all of which must be considered and mitigated when using the tools.
1. Sensitive IP Disclosure
The first significant risk is that users will provide sensitive data to AI tools like ChatGPT, which, in turn, will store and use that data in ways that may be unacceptable to the organization. For example, tools like GitHub Copilot must analyze your (potentially sensitive) source code to provide programming suggestions. To generate a response, the generative AI tool must receive and process data. But how is that data stored? Can it be deleted by the user on demand, possibly to comply with regulations like GDPR? Will data submitted to the model within prompts be used to retrain later iterations of the model?
As of the writing of this piece, OpenAI does not use data submitted when using ChatGPT’s enterprise plans for improving its models. But data submitted to OpenAI’s consumer plans—including the paid ChatGPT Plus offering—is available for use in retraining the model. This means sensitive data sent to the consumer versions could be regurgitated to users outside the organization at some point. This isn’t happening yet with ChatGPT, because the models are not being updated in real time. However, nothing legally prevents OpenAI from using the data in this manner.
2. Ownership of Generated Data
A common use of generative AI tools is to help create content, such as code, images or written copy. However, there are significant unresolved legal questions about the ownership and use of the output:
Several IP creators have sued generative AI companies, alleging inappropriate use of creator data to train the AI models: For example, some developers sued OpenAI and GitHub over the use of their data in coding models (including GitHub Copilot and OpenAI Codex). Plus, Getty Images sued Stability, the company behind the Stable Diffusion image generation tool, for alleged license violations, claiming Stability used Getty’s image set for training its model.
While OpenAI has not yet been sued over ChatGPT as of this article’s original publishing, it could be a possibility as OpenAI has not revealed the sources of its training data and it is unclear which parties might have standing to do so. The only major public generative AI tool to assert ownership or license over its full training data is Adobe with its Firefly image generation product.
The U.S. Copyright Office issued guidance in March 2023 saying works generated by AI cannot be granted copyright: The guidance indicates that while a prompt (the data submitted as an input) could be granted copyright, the output could not be.
3. AI Hallucination Impacts
AI “hallucinations” are defined as generative AI outputs that are mathematically sound according to their model but provide factually inaccurate data. There are numerous public examples of LLM hallucinations that would create liability for any organization relying on them. Hallucinations in AI models are not going away. They are a feature, not a bug.
4. Bias Impacts
Bias in the training data of generative AI and LLMs may also affect their output. For instance, consider the prompt term “to boldly go.” If you’re a Star Trek fan, you “know” what should come next (“where no man has gone before”). But if your model wasn’t trained on any Star Trek data, you would likely receive a much different answer. Likewise, consider if you aren’t looking for anything to do with Star Trek. Due to the TV show’s popularity, however, it may have the majority of entries in the training data set with the trigram “to boldly go.” You’ll end up getting responses from science fiction, potentially without knowing it. Most users don’t understand what’s in the training data for the LLMs they use.
8 Tips for Using Generative AI Securely
To reduce the likelihood of generative misuse scenarios and security risks of generative AI adversely impacting your organization:
- Review third-party service agreements with generative AI in mind: Have your legal team review your contracts and agreements to determine how generative AI tools might impact the safety of your data. Be sure to include provisions that limit fourth-party risk, such as when a third-party service transmits your data (knowingly or otherwise) to a generative AI tool.
- Classify data with generative AI in mind: Most organizations have regulatory and contractual agreements governing data usage, and these obviously must be adhered to. But most source code within an organization is not sensitive, and developers might gain some advantage by using generative AI to optimize or debug their code. Traditionally, there was no benefit to creating separate data classifications for data that should not be publicly shared but can be shared with a third-party for some benefit. Generative AI tools change this calculus and may justify the additional data classification work to get the most out of the tools.
- Check with legal counsel on output ownership: As noted earlier, there are significant concerns in the legal community about the ownership of data generated by AI tools. The U.S. Copyright Office guidance provides additional cause for concern. Consider, for example, how its guidance might impact code generated while using GitHub Copilot. Can your developers assert which code they wrote (so it can be copyrighted, including the prompt for generated code) vs. code Copilot generated (which is not eligible for copyright)? Organizations using generative AI should discuss ownership of data generated and how use cases might expose the organization to risk while legal cases remain pending.
- Identify acceptable and unacceptable use cases for generative AI, rather than fully allowing or blocking: Use cases should be evaluated by members of the security team familiar with the risks of AI, the risks of the use case documented and any controls for mitigating risk implemented.
- Educate users on the generative AI use cases the organization deems acceptable: You should also explain why other use cases are not approved. By sharing the decision logic, the organization can minimize the number of potential use cases that need to be risk assessed.
- Implement guardrails to protect against hallucination impacts: Don’t confuse this recommendation with identifying acceptable use case. While no use cases where hallucinations would have catastrophic impacts should be approved, guardrails (often process-based) expand the number of use cases where the risk is acceptable. For instance, consider requiring a senior analyst to approve an AI-derived course of action.
- Establish safe harbor policies for employees using generative AI: As more organizations turn to generative AI to enhance productivity, we are likely to adjust what we see as “acceptable” output levels from employees. With expectations and pressure increased, users will start failing to check the output of the generative AI, because doing so would largely negate productivity gains. Because hallucinations are a feature of generative AI, such users will eventually create risk (or even direct harm) for the organization as a result. Organizations should create safe harbor policies for the use of these tools or risk wrongful termination or other claims from employees if adverse action is taken against them as a result of their AI use.
- Revisit these guidelines as generative AI evolves: This is a highly dynamic technical space and these recommendations may change over time as more technical controls become available.
Although reasonable efforts will be made to ensure the completeness and accuracy of the information contained in our blog posts, no liability can be accepted by IANS or our Faculty members for the results of any actions taken by individuals or firms in
connection with such information, opinions, or advice.